Four Steps To Keep Data Scientists In Their Happy Place
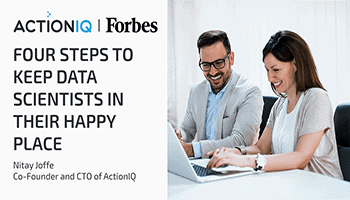
Data scientists love the analytical work of building and testing new algorithms. Data wrangling—the work of hunting for, validating, connecting, and combining data sets—not so much.
In a new article published by Forbes Tech Council, ActionIQ’s co-founder and CIO Nitay Joffe writes about his experience as a software engineer at Facebook, where faced exactly this challenge. It was his job to unify predictive scores with customer profile information in order to deliver ads in an optimal way.
“I kept asking myself what capabilities would be required for data scientists to locate the latest, greatest data instantly and start modeling it right away,” writes Joffe.
That question helped inform the architecture of the customer data platform he helped design for ActionIQ. Here are the principles Joffe says are required to help keep data scientists in their happy place:
- Gather data instantly. This requires the ability to ingest raw, granular data without complex ETL processes. However, there must be some kind of order to the data. For marketing, that means making customer IDs the prime logical unit, with a clearly defined set of data types that that help define individual customers.
- Validate data instantly. Data types are constantly changing, though these changes are often not at all transparent to data scientists. This can makes the data validation process grueling. However, you can avoid this risk by creating a separate layer for defining the business rules for attributes, this requiring no changes to the underlying raw data provided by source systems.
- Connect data instantly. Data science necessarily involves working with data from disparate systems that use disparate data models. You can dramatically reduce the complexity of combining data by dividing organizing customer data along two essential dimensions: 1) attributes that define a specific customer, e.g. email or physical address; and 2) behaviors, such as purchases, store visits, web browsing history, etc.
- Select data on the fly. Data scientist involves a process of trial and error in which you test various combinations of data types, a process known as feature engineering. Again, when can keep the definition of attributes in a separate layer, you make it easy for data scientists to select any set of variables they want—and keep changing and iterating that selection on the fly, until they achieves the results they are after.
When you can remove the burden of data wrangling, writes Joffe, “You don’t just remove the cost and complexity of data prep; you also unleash the creativity of data scientists, making them both happier and more productive.”
Read the full article in the Forbes Tech Council.