3 Reasons Out-of-the-Box Artificial Intelligence Falls Short
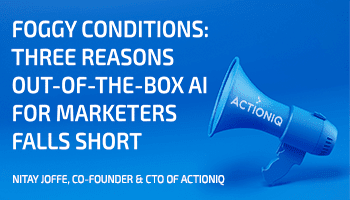
To unlock value hidden in customer data, brands have been adopting Artificial Intelligence (AI) solutions at a breakneck pace. However, if your brand is relying on out-of-the-box algorithms, you may be missing out on the true value of AI—i.e. the hidden insights that only your own unique data of your unique set of customers base can provide.
In a new article published by the Forbes Tech Council, Nitay Joffe, co-founder and CTO of ActionIQ, explains why out-of-the-box AI may only take you part way to your destination—and could even lead you in the entirely wrong direction.
Of course, the speed and convenience of out-of-the-box AI can be tempting. By limited yourself to a fixed set of data types, you speed data prep. Nor do you need data science resources specialists to create or refine algorithms.
The problem is, these pre-existing algorithms grow out of the data of other brands, not your own. That means you are stuck with:
- Lowest-common-denominator data. Instead of drawing on all the rich data your brand collects, you are limited to those basic data types that every brand collects and has within reach.
- Black-box algorithms. When you have no idea how, for example, a churn risk model is built, you have no way to evaluate its efficacy, much less optimize it for your brand’s unique customers, offerings, and brand strategy.
- No learning or results-based refinement. AI algorithms are meant to learn from their own mistakes—measuring the accuracy of their findings and “self-correcting” accordingly. That is impossible when you are limited to a black-box model.
Sure, out-of-the-box AI can provide a certain degree of value. “But it is critical to understand its limitations and consider embracing AI’s true promise — insights that grow directly out of the full spectrum of your unique set of data,” concludes Joffe.
Read the full article at Forbes Tech Council.